The new competitor, generative AI, and the new hope!
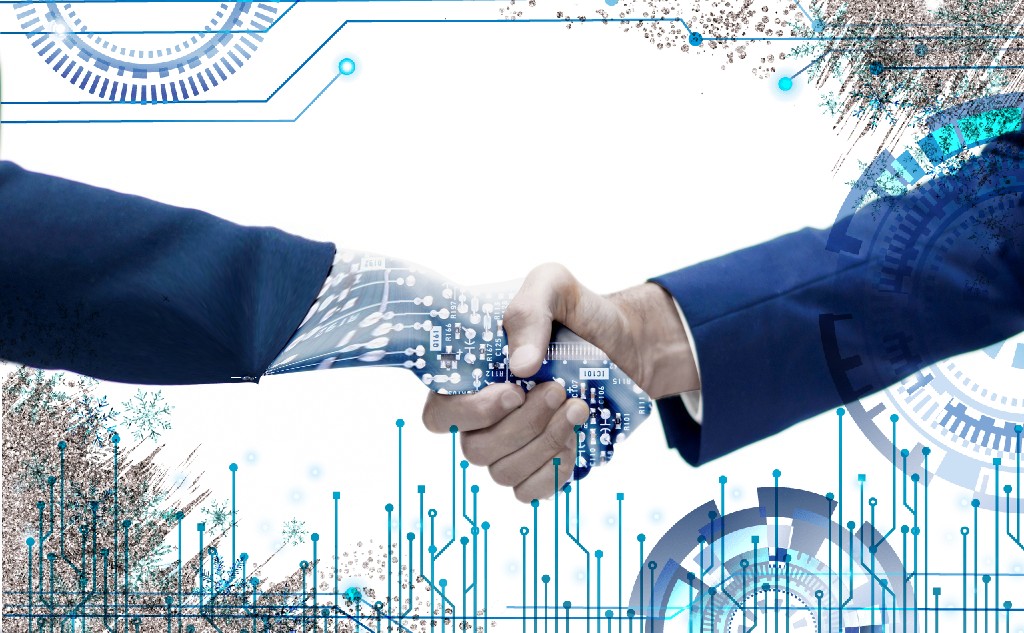
The new competitor – generative AI.
The new competitor, generative AI, has arrived. Undeniably, generative AI has changed the way many of us work. This time, it is directly impacting the functioning of IT companies, marketing offices, back offices, etc. Certainly, the launch of chatGPT has accelerated the release of many competitive generative AI-based tools and platforms. However, this time, the competition and the impact are going to be very different. As AI startups create tools to create source code and tests, more such tools and platforms are entering the market. Due to this, large IT companies have to compete not only with peer-sized companies but also with smaller AI startups and more in-house software development. The competition is shifting towards (1) size of the company vs. output, (2) Energy efficiency vs. labor cost, (3) Original vs. fake, and (4) Proprietary vs. Open Source. These changes are creating a shift in the job market and skill requirements as well.
Current Model of Development Vs New Model of development
The race to adopt generative AI
There is a race to create various generative AI models. There are two kinds of markets that are evolving.
(1) Adopting open generic models: Adopting open source as part of mainstream work We are getting into a situation where there will be a number of standardized open-source generative AI building blocks that can be easily integrated into mainstream work. In fact, these kinds of blocks have evolved over many decades. However, the scale of changes due to generative AI is significant. Because of these tools, the potential to create a large scale of output has vastly increased.
(2) Domain-specific or proprietary models: Though there are generic models, there is a need to develop domain-specific and context-specific models. For example, in the medical field, treating diabetes is related to the pancreas and lifestyle changes. Treating cardiovascular diseases is a separate domain by itself. These models cannot be generic. These are not only domain-specific but also more complex problems to solve.
Creativity at ease!
In artistic and creative environments, the possibility of creating multiple options is much easier. For example, for a movie costume, instead of physical costume creation, we can use generative AI models. This will help reduce the cost of film and drama production. Most importantly, it will reduce the waste of non-usable products.
Creating a more intuitive user experience: For example, the user interface of an application for a given generative AI model can create multiple UI options. If these tools are integrated into requirements management itself. A better UX will be created by many applications. This way, the reduction in time spent creating a user-rich experience will be enhanced.
Better workflows for applications: we have experience using many applications where we have to navigate like a maze. Generative AI can help reduce user workflows! By doing so, the focus can be moved to a rich user experience rather than a rich UI.
Opening new doors: Consider the restoration of old paintings and architecture. According to normal methodologies, this will require an enormous amount of time and effort. Now we will have more options.
Productivity Reimagined
Imaging tools and Diffusion models: These models give output as images or videos. The launch of Adobe FireFly has created a buzz in the market, especially with marketing people. With prompting, the background of the picture can be changed seamlessly. Creating marketing content becomes much easier. Productivity is unleashed, but much creativity is also not required! While that has created hype, there are other similar tools like StyleGANEX that have become alternatives in terms of creating image or video toons with reference images, etc.
Coding and test tools: Codium is one of the code-generating AI tools. Currently, it integrates with the IDE. However, there are other software engineering tools that can help code and test. Microsoft’s GitHub Copilot is one of the code-generation tools. The advantage is that it is estimated that there are more than 100 million developers using this tool. Hence, this becomes a strategic platform. The users in the community will be more open to using it and sharing it. Contrary to Copilot, Tabnine helps run the AI tool in the local environment and keeps the code secure. Another interesting tool that helps to reengineer the existing code base is Sourcegraph. MutableAI is yet another GPT-based code acceleration tool.
Multiagent frameworks: MetaGPT and Mava are multi-agent frameworks. There are many multi-agent frameworks. These frameworks provide agents based on the role. Project Manager, Programmer, Test Engineer, Architect, etc. Based on the role and objectives of the application, the product source code is generated! This will drastically change the ways of working of a Project Manager or any other different role.
Challenges of using generative AI
- Integration: How to integrate generative AI in legacy systems, in an embedded software environment, or in a custom architecture environment To integrate, additional resources and time are required to train custom generative AI models. This is going to be one of the challenges.
- Open Source: Every organization cannot create its own models. However, these models are to be customized and deployed locally.
- Security: Even by mistake, these models can create malicious code. In that case, How to Limit the Power of Generative AI
- Limiting the usage: how to limit the usage of generative AI only in a limited environment For example, how do I make the models only for business purposes and for the intended purpose only?
Destruction to Disruption
On a short term, there are are some adverse impacts of the the generative AI, however by careful measures, these adverse impacts will start impacting positively
Short-term destruction | Long-term benefits |
|
|
|
|
|
|
|
|
Two missing senses of generative AI
- Enterprises, big and small, are in some way adopting generative AI models. Broadly, it is classified as “Speech”, “visuals,” and “Audio” . Speech and Audio are challenging. There are dialects and pronunciations, and it is estimated that there are more than 7000 languages in use. That’s why prompting is a much easier interface to work with generative AI. The input is almost precise. As NLP has evolved over a period of time, its accuracy has increased significantly.
- What about other senses like touch and taste? How generative AI can play a role using these senses. The most important challenge is, without using any additional devices, how generative AI can be creative in
New opportunities and New hope
-
Most of the generative AI is currently running on the cloud, and in the future, some of it will have to run on devices as well. However, most of the GPUs are running on the cloud, and some of the emerging jobs and hopes
- Integrating generative AI models in a secure way requires re-architecting some of the existing systems. Implementing large enterprise systems like SAP, Oracle, or MS Dynamics is not straightforward. Hence, the need for customization, integration, and changing the workflow arises. Therefore, it creates a new kind of job. Moreover, this is still in its infancy. As of now, companies are trying to train their engineers to become familiar with AI. As the implementation grows, we will figure out the kind of training required.
- Already, the need for a new kind of database has come up. In a vector database, there is semantic information in the database for understanding the context. All these require a new kind of job. But we will be doing it in a smarter way.
- Basically, we are moving towards “AI Adoption Architects and Engineers” or “AI Implementation Architects and Engineers/Security/security/ethics” and so on. The game is shifting from developing generative AI models to “adopting and implementing”. This requires a completely new way of adopting the AI models, which will require a massive transformation.
- Since we are in the initial phase, it is still evolving, but from my viewpoint, there is a new hope of new jobs and new ways of working.
Preparing now for the future
-
We cannot resist the adoption or impact of generative AI. Whether we like it or not, generative AI is proliferating. There may be many challenges to adoption, but the race has already started.
- Start now. This is the time to start. Not tomorrow. Now is the time to start
- Impact analysis: In the area of service, it is important to assess what areas generative AI is going to impact. Some may be due to automation, and some may be alternatives or new ways of working. Acknowledging this impact is very important.
- Opportunity analysis: Understanding the ecosystem creates a new kind of opportunity. For example, to modernize a legacy CRM system using generative AI We may have to retain the core engine for various reasons, but use generative AI for dashboarding and reporting. Identifying opportunities at an early stage is important.
- Reskilling: the Reskilling of existing employees to embrace the models There are going to be a plethora of models floating on the market. Intelligently choosing the right models will make a difference. Architects may have to learn how to use architect agents so that they become more generative AI-friendly architects.
- Adopting new skills: Freshmen can make a leap forward in learning new concepts. However, this is going to be more self-learning. The gap between employability and education will further widen. However, microlearning aspects will help further accelerate
Yes, while there are threats, new kinds of opportunities keep evolving. Certainly, we are in the middle of disruption and big changes. However, an opportunity for us to create a positive impact in this world emerges. In the end, generative AI models will keep opening new doors and opening up new fields in an unprecedented manner.